(*) I wrote this article in Medium in 2020
Curiosity is a fundamental part of every Designer’s personality. As User Experience (UX) Designers, finding the best way to meet the users and the business needs is our main goal. However, “the best way” should not be the most trendy or pretty one, but the most efficient one. Researching and understanding Artificial Intelligence (AI) capabilities enables UX Designers to create AI-powered proposals with a human-centred approach, as well as boost our workflows.
“Design is not just what it looks like and feels like. Design is how it works.” Steve Jobs
AI or not AI
AI plays an extremely important role in improving the users’ experience by providing disruptive and much more efficient solutions. However, many companies are forcing their teams to introduce AI features just because it is a buzzword, without understanding its capabilities, and if it is worth investing in it. Introducing AI must be a decision based on the belief that it is the best way to improve the product. A problem should not be created to introduce AI. AI should be introduced as the best way to solve a pre-identified problem. In addition, many Product Owners and Designers face challenges in understanding how AI works and what it can provide. So, even though they identify a problem that could be solved with AI, they do not follow that path due to a lack of knowledge.
Based on this, let’s first have a high-level look at what AI, Machine Learning and Deep Learning are:
1/ AI
AI is the broadest way to think about computer intelligence. It can be associated with anything from a voice-recognition system to a playlist recommendation based on your interests. The technology can be categorized into two main groups: artificial narrow intelligence (ANI) and artificial general intelligence (AGI).
- Artificial narrow intelligence (ANI): It is where we currently are. Narrow AI can perform one specific task. Apple’s face recognition system, a computer playing chess or even a self-driven car are all examples of ANI.
- Artificial general intelligence (AGI): It is where we are going. This type of technology would own the cognitive abilities to understands the world as we do and can perform a range of tasks. AGI is considered “human-level”.
Some people consider a third group called Artificial superintelligence (ASI). Nick Bostrom, Oxford philosopher who popularized the term “superintelligence” defines it as “an intellect that is much smarter than the best human brains in practically every field, including scientific creativity, general wisdom and social skills.” The technological singularity is a hypothesis predicted on the creation of artificial superintelligence. This would trigger a kind of tipping point in which enormous changes take place in human society.
2/ Machine Learning
Machine learning is a subfield of AI where machines take data and “learn” for themselves improving their responses. ML algorithms find patterns in massive amounts of data, and then use those patterns to make predictions such us what type of show you might like. There are two types of Machine Learning:
- Supervised learning: The data is labelled to tell the machine exactly what patterns it should look for.
- Unsupervised learning: The data is not labelled to let the machine look for whatever patterns it can find.
2/ Deep Learning
Deep learning is Machine Learning on steroids and it uses Artificial Neural Networks. It consists of several artificial neurons connected to each other to simulate the brain’s function delivering a final result in the form of a prediction. The higher the number of neurons layers, the deeper the network is. In this case, we add a third option of learning:
- Reinforcement learning: Learns by trial and error to achieve a clear objective
Perfect Couple
Now that we have a high-level understanding of what AI stands for, there is no doubt that it can add value not only to a company’s portfolio, but also to its workflows. Both Data Scientists/Analysts and Ux Designers jobs can be enhanced by working together:
AI to improve UX processes
The UX Design process follows a Design Thinking approach which is based on 5 phases. AI can be used to make this process much more efficient delivering better outcomes:
1/ Empathize: The key to empathize is to understand, and to understand we need to learn about the audience.
[AI to improve Research] UX Researchers collect huge amounts of data. Analyzing it takes a lot of time and it can be a very complex task. AI can find patterns based on users behaviours that would be difficult to identify by a human. It can also cluster users to build segments and to understand what binds them together.This will optimize the UX Researcher work and let the UX Designer provide more meaningful prototypes as well as gather better feedback minimizing product errors.
2/ Define: Identifying the users' needs and prioritizing them based on the business goals and the value proposition.
[AI to customize experiences] Some products have a wide range of user types. AI could detect profiles and past behaviours to select from a set of experiences and provide the most intuitive and positive one for each particular user.
3/ Ideate: Generating ideas for the design.
4/ Prototype: Turning ideas into concrete examples.
[AI to move ideas to reality] Some existing tools are able to recognize sketches and transform standard hand-drawn designs into high fidelity mockups or source code. This allows UX Designers test high fidelity ideas much more quickly (Ulizard / Airbnb AI)
5/ Test: Evaluating the design. Iterate.
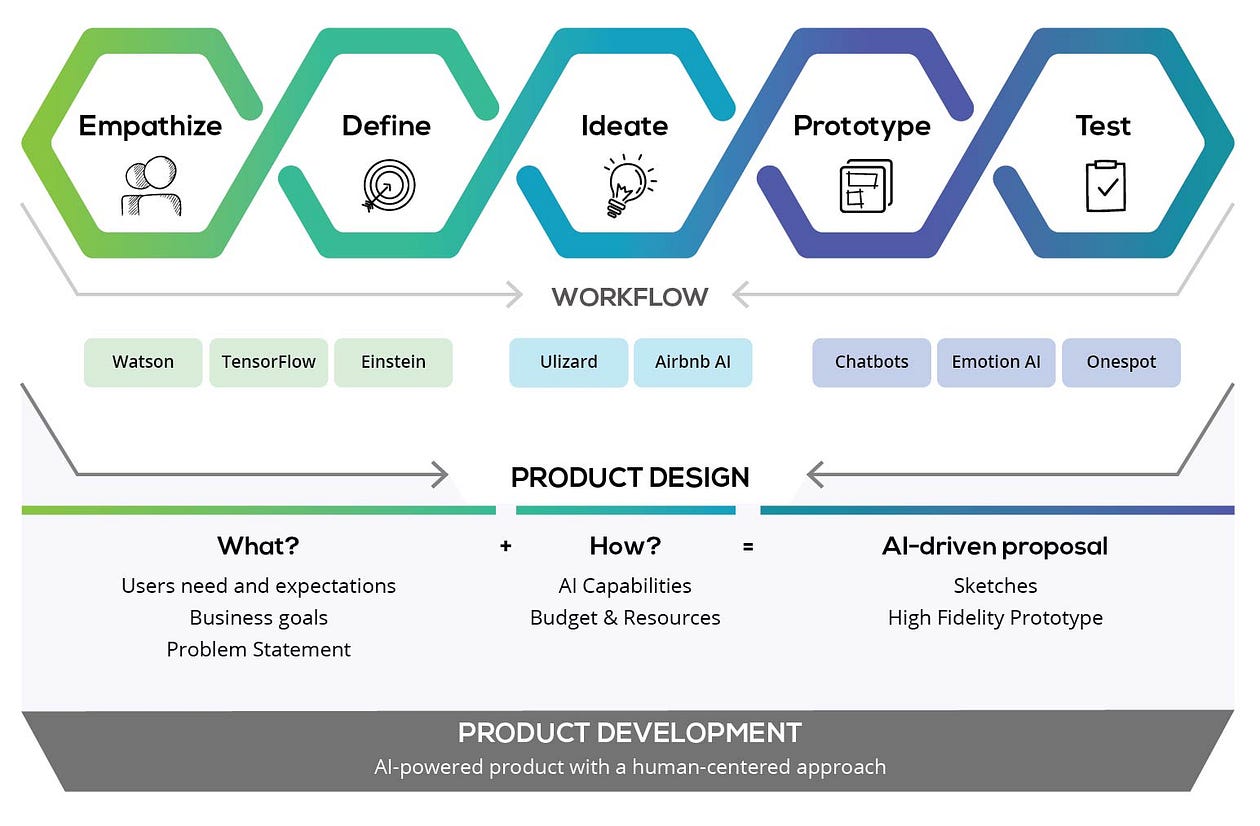
UX to create meaningful AI-powered products
It is important to highlight that introducing UX early on in a product development cycle helps to anticipate, mitigate and reduce errors which saves costs down the line. Fixing a problem in development costs more than fixing it in design, and much more if the product has already been launched. In addition, recovering a frustrated customer can be a really hard task.
Also, UXers engaged in ongoing collaboration with Data Scientists help to envision what to make and to define priorities based on the users and business needs. The combination of both skill-sets helps to move from a data-centric culture to a user-centric culture that leverages data to enhance the experience.
1/ Empathize and 2/ Define: Before introducing any AI capability, we first need to understand the users' needs and expectations to identify the real problems.
3/ Ideate: With the problem statements previously defined, the team can sort them into categories (f.i: Analytic, Functional, Interactive, Content) and start answering questions such as: Would an AI-driven solution solve a real user need? Would it be the best way to solve it for both the users and the business? Is it worth investing in it?
A few examples of AI solutions for each category could be:
- Analytic: Risk assessment, sentiment analysis, retroactive analysis
- Functional: IoT solutions, robots, mechanical apparati
- Interactive: Personal assistants, chatbots, Google Home, Alexa
- Content: Natural language processing, text recognition, speech-to-text conversion, Computer vision, augmented reality
4/ Prototype and 5/ Test: By creating sketches, mockups and animated prototypes, the team has the chance to evaluate the idea, collect feedback, and improve the proposal before investing too much time and money on developing the end solution. A team that creates an AI-driven solution based on user research and testing is the best and most efficient way of improving a customer’s experience. Utilizing this approach is much more likely to produce a solution that satisfies both user needs and business objectives.
Conclusion
AI has increasingly become a strategic focus for many businesses. This strategy is certainly a wise investment. However, AI efforts will likely fail to deliver on anticipated value unless the benefits of AI are focused on solving real customer needs that improve their overall experience.
Currently, many UX Designers overlook simple opportunities to include AI in their projects because they face challenges understanding AI capabilities. On the other hand, many Data Scientists and Analysts focus only on the datasets without a deep knowledge about the users pain points. A multidisciplinary team consisting of both UX Designers and Data Scientists/Analysts is crucial in defining when and how to enhance a product by leveraging AI. They have the perfect skill-sets not only to help the company save money by mitigating errors, but also to move it to the next level with solutions that follow the latest trends in the market.
References:
- Introduction to Chatbots | José Angel Rodriguez Ramos — EY
- Introduction to Neural Networks | Juan Ignacio Mazza — EY
- Reimagining the Goals and Methods of UX for ML/AI — Philip van Allen
- A project guide to UX Design — Russ Unger, Carolyn Chandler
- What is machine learning? Karen Hao
- What is AI? We drew you a flowchart to work it out — Karen Hao
- The Designer’s Guide to AI-Driven UX